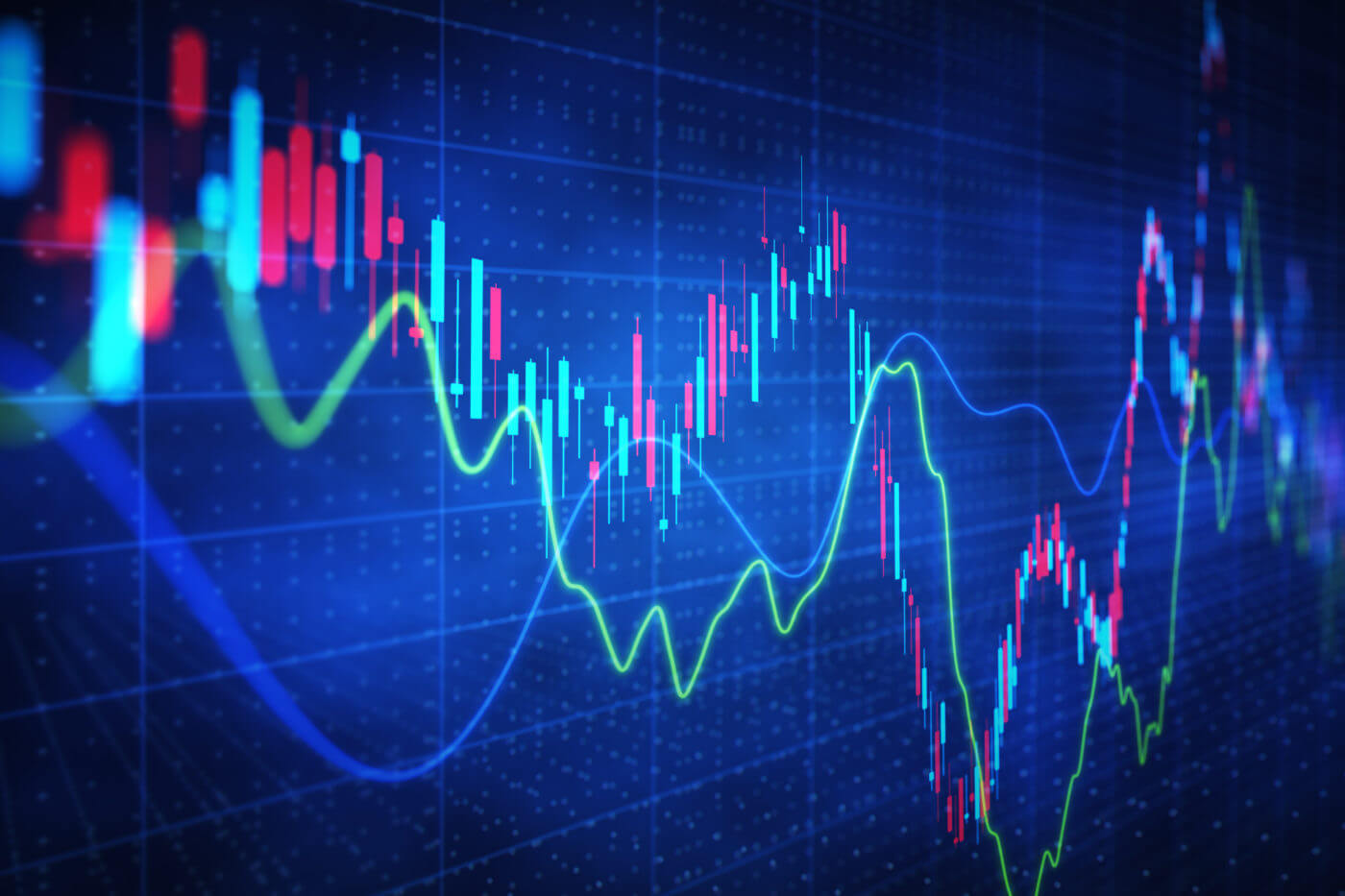
Always wrong, sometimes useful: 5 Tips for understanding the value and limitations of decarbonization models
Models are often at the center of discussions around climate change, mapping out highly uncertain future scenarios to help decision makers understand what needs to be done, by when.
Models provide a concrete basis for policy creation, providing comparable scenarios that address the vast array of options and pathways that are on the table. Almost as importantly, models also help the climate community construct accessible narratives about possible future outcomes. They serve as a sort of tether for all the conversations and arguments in the climate community, which is why you are likely to hear about the need to “keep warming below 1.5 degrees Celsius” from an advocate, an elected official, an academic, or a policy analyst.
The problem is that models are inaccurate.
Most energy system models that inform policymaking and advocacy efforts at all scales — from local to global — are deterministic, economic optimizations of which the outputs are a handful of improbable scenarios. These models are referred to as “black box” models because there are many assumptions hidden within them that are difficult to discern. They are based on limited information and built around assumptions or constraints designed to make the complexity of reality easier to predict. The problem is, there is always going to be a difference between ‘model world’ and the real world because, fundamentally, human beings cannot predict the future.
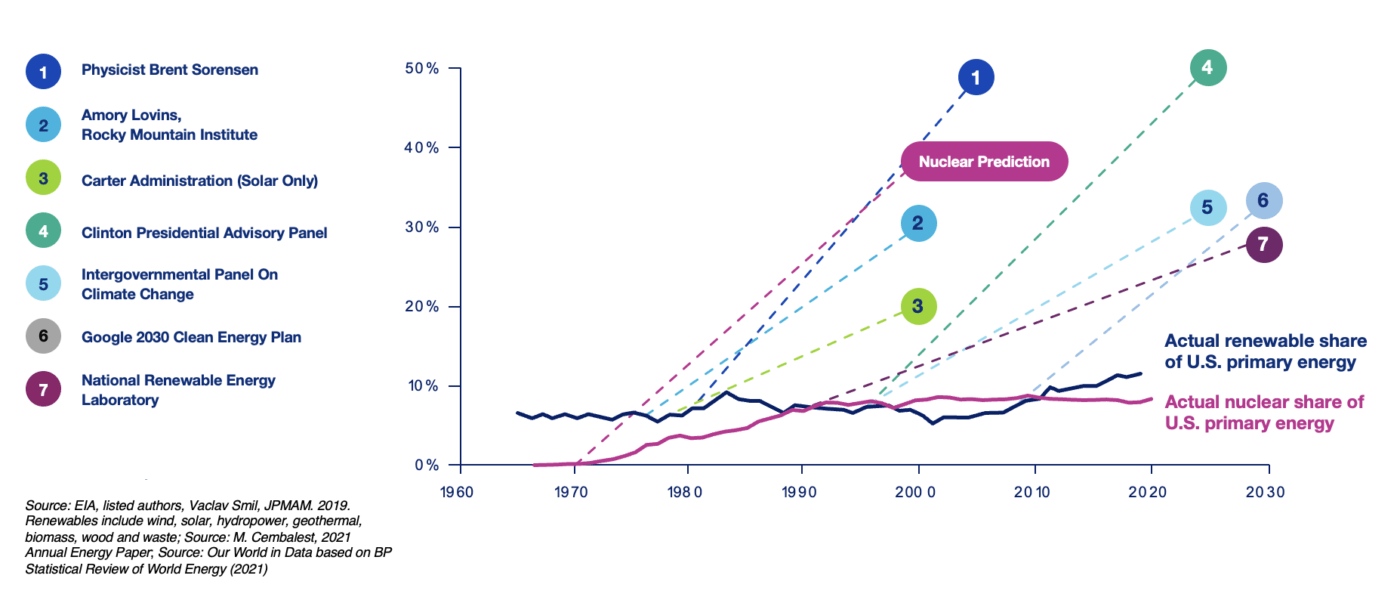
1. Finding the lowest-cost solution is not the same as finding the best solution
Energy system models are commonly designed to find a solution that achieves net-zero emissions by a certain time for the lowest cost. However, these costs are based on highly uncertain projections and do not include indirect and difficult-to-monetize costs and benefits of an energy transition, such as human health improvements, equity implications, and environmental impacts. As a result, these ‘least cost solutions’ may not actually minimize the costs — let alone maximize the benefits we as a society prioritize.
For example, a least-cost solution may achieve net-zero emissions in 2050 by allowing unabated fossil fuel use until 2049 before switching to a more costly zero-carbon source of energy, achieving an ultimate net-zero emissions objective. Under this scenario, however, heat trapping gases will build up in the atmosphere up until 2049, increasing warming for centuries and failing to achieve broader climate goals.
In the figure below, the gray represents natural gas installed capacity and the dotted line represents cumulative emissions. While both panels show natural gas reaching zero by 2050, the pathway towards achieving this goal has different implications for cumulative emissions. On the left, 2050 cumulative emissions are high because natural gas continued unabated until a rapid switch to low– carbon resources near 2050, while the figure on the right shows lower cumulative emissions due to a steady transition away from fossil fuels.
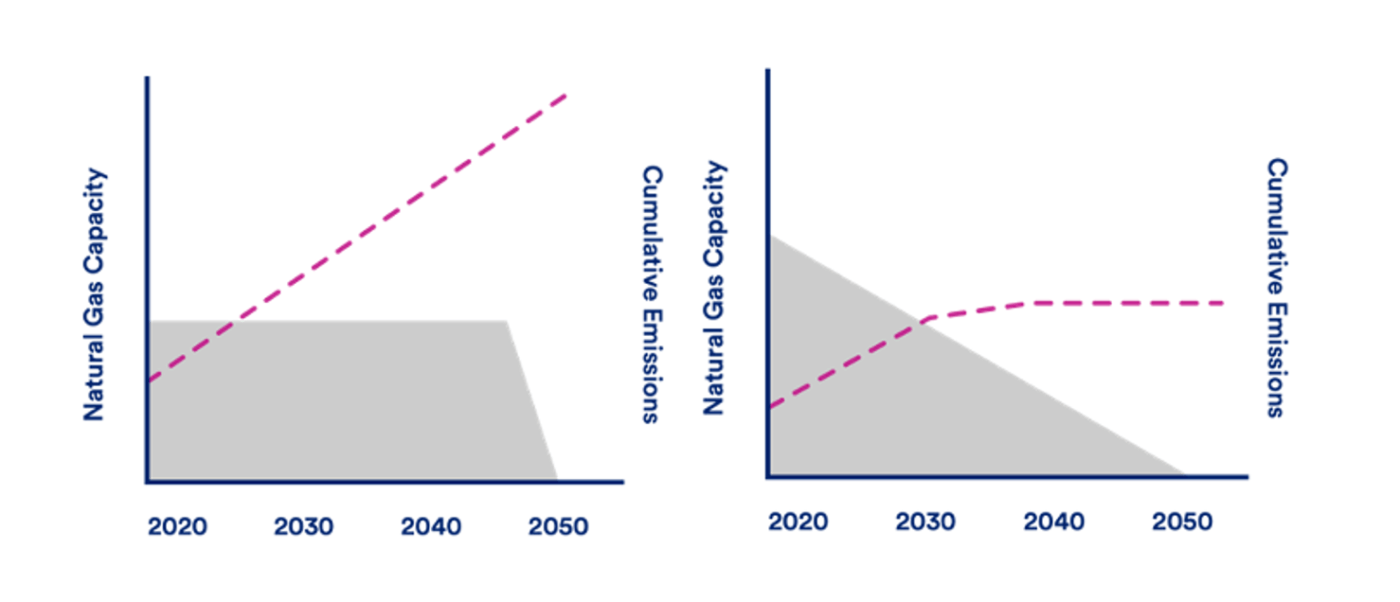
When reviewing energy model results, be sure you understand what is being minimized and how this aligns with your broader values and objectives. Also be sure to review the cost assumptions, which often can differ between organizations and can reflect their inherent bias towards different technological solutions — if you disagree with the included projections about renewable costs for example, you should be cautious of accepting the model result that are based on these cost estimates.
2. We need to decarbonize the entire economy, not just the electricity sector
Even with a high degree of electrification (e.g. transitioning to electric vehicles) and energy efficiency measures, the electricity sector is projected to represent only about half of energy demand in 2050.1 Non-electricity energy demand, in the form of carriers like fuel, will continue to come from the industrial, transportation, commercial, and agricultural industries. Because these so-called ‘hard to abate’ sectors — for which decarbonization strategies are more difficult and technological solutions immature — are nearly exclusively non-electric, models that only account for decarbonizing electricity demand will fail to reduce emissions enough to meet climate targets.
For example, if an electricity sector model does not account for electrolyzers that generate zero-carbon fuels like hydrogen that could contribute towards decarbonizing the production of cement and steel, the projected future demand will be underestimated. Similarly, if carbon removal is not considered in the electricity sector model but is required to decarbonize the economy, projected electricity demand will also be underestimated.
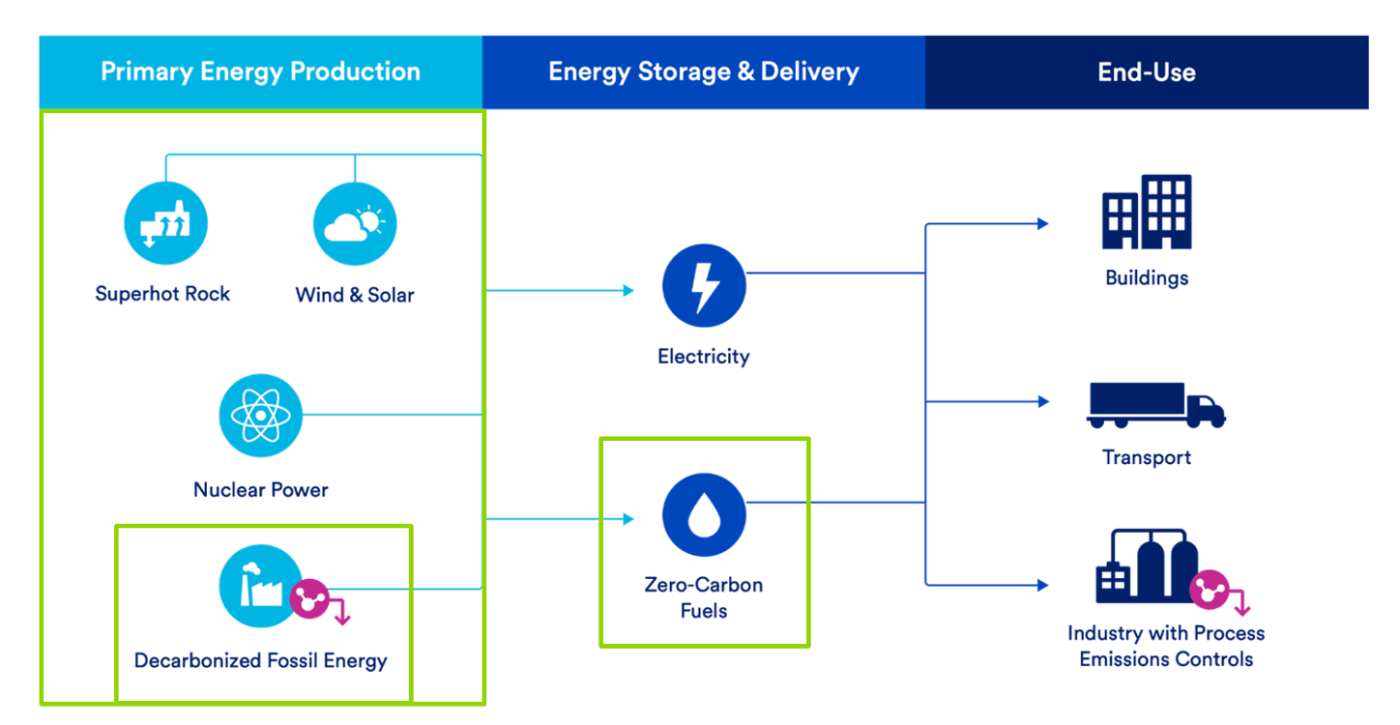
When reviewing energy model results, be sure you know what sectors are included in the modeling and how the sectors are related to each other. ‘Economy-wide’ models seek to decarbonize all sectors, while ‘electricity-sector’ only models focus exclusively on decarbonizing the electric grid. Again, an optimal electricity sector or even energy system solution is likely to be different than an optimal whole economy solution. Ensure the type of model is aligned with your purpose in using the model results.
3. The reliability of an electricity system is often undervalued
As electrification increases, power outages are expected to have greater societal impacts. Decarbonization model results, however, typically do not discuss reliability of a projected energy future, even though people have come to expect reliable access to electricity in many places around the world
Here are some key indicators to consider that may reveal reliability concerns:
- How many “weather years” does the model use? Weather years refers to the weather data that is included to determine the renewable electricity output, along with energy demand for things like heating and cooling. Many models use a single past weather year to model each time period to 2050. This may lead to an unreliable grid, both because weather has high annual variability and because weather patterns are likely to be different in future years due to the effects of climate change.
- How is load balancing done in the model? The operators of electricity grids balance supply and demand down to fractions of a second, but due to computational limitations, modelers face tradeoffs in simplification of the system versus accuracy. Oversimplifying grid balancing hides important demand variations. For example, the capacity needed to meet the average daily demand would not be enough to meet peak daily demand, and would thereby result in an undersized energy system. This is especially problematic because one of the biggest decarbonization challenges in the electricity sector is consistently and reliably meeting peak demand. A common practice is to balance supply and demand on an hourly basis, known as ‘8760 modeling’ after the number of hours in a year.
- How reliant is the model on demand flexibility? Demand flexibility refers to shifting load to make demand match electricity supply at peak times of the day. This can include charging EVs at night instead of during the day, shifting air conditioning to different hours of the day, and reducing refrigeration for some commercial/industrial customers for short periods of time. A high reliance on demand flexibility may indicate the modeled grid could be less reliable due to a consistent daily mismatch in supply and demand.
When reviewing energy models, look for key indicators that suggest how reliability is embedded or excluded from the modeled system. You should not take for granted that a model result would operate with high reliability and zero blackouts.
4. Model scenarios are not predictions of the future
Model results are presented as scenarios that represent one way the future can unfold. For example, one scenario may show electricity sector decarbonization through 100% renewable energy only, while another may represent a future with renewable energy and zero-carbon fuels. While scenarios are intended to reduce complexity, these scenarios are just stories of how the world may end up and are not rooted in likelihood or risks. Because they may be compelling, they can cause us to overlook a wide variety of more likely outcomes and lead to overconfidence in decision making.
Scenarios should also be interpreted cautiously because they do not facilitate risk-informed decision making. Beyond failing to identify the likelihood that a specified future will occur, scenarios do not describe the consequences of being wrong. This does not allow us to make decisions that minimize regret of the actions we take and maximize likelihood of successful decarbonization. Instead, because it is difficult to know which scenarios are more likely to occur, we should be looking to see what decarbonization pathways can be successful across a range of scenarios; rather than guess what future we will face, we should plan to decarbonize successfully under a variety of futures.
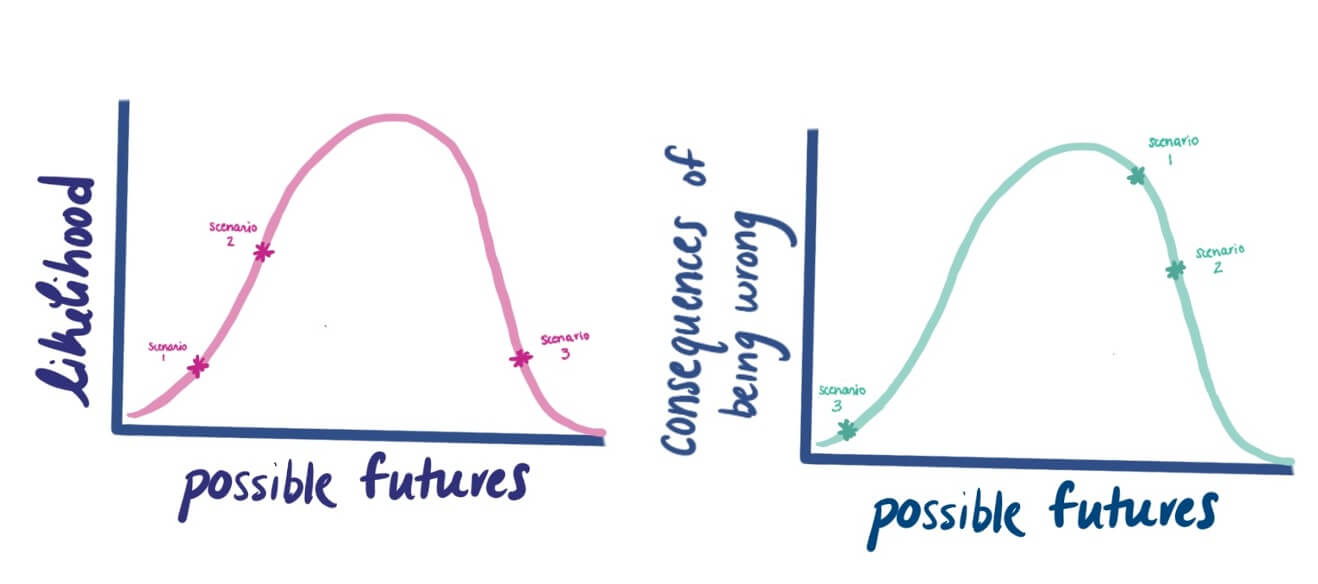
When using model results, be sure to understand that the scenarios portrayed are just single examples that illustrate one possible version of the future. Do not conflate a scenario result with a prediction of a likely pathway, nor as a risk-informed solution to mitigating energy emissions. Think critically about the degree of transparency in the scenario design and the motivation behind scenario selection. Be wary of modeling efforts that draw conclusions based on a single scenario or make recommendations according to a limited set of future stories.
5. Models should not assume miracles
Gaging plausibility is a crucial step in critically reviewing outcomes of a model.
Plausibility, or lack thereof, can come in many forms. For example, model results may show hydrogen fuels replacing natural gas in power plants over just a few years’ time without describing how or when the hydrogen pipelines or other logistical means of hydrogen distribution would be established. Additionally, models can be based on the assumption that the developing world will remain at low levels of energy consumption, when in reality it is plausible and morally desirable to assume energy consumption per-capita will increase. As a third example, model results may show electricity trade across states, countries, or regions without accounting for the real-world challenge of siting, funding, and building an expansive transmission network. Other common examples are assuming unrealistic costs (or omitting certain costs all together), impractically short timelines to introducing new infrastructure (without regard for historical data or supply chain limitations), and high levels of consumer participation in decarbonization policies (like demand flexibility
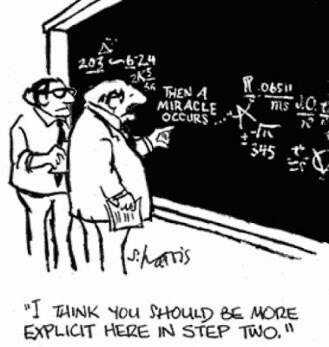
When evaluating model results, be cognizant of the feasibility of implementation and the plausibility of input assumptions. Question the necessary enabling conditions that were excluded from the model and evaluate the feasibility that those can technically and economically occur within the necessary time frame. Finally, check that the assumed social context that enables the model outcome to successfully decarbonize is plausible as well.
Making better models
We do not only need to learn how to evaluate existing climate models more critically; those within the modeling community must also shift away from traditional optimization towards holistic analytical approaches to hedge risks and prioritize systems resilience. Through better disclosing the implicit assumptions in modeling and planning, improved climate modeling can provide policymakers with the tools to support a more feasible, reliable, and socially responsible energy transition.
The Energy Systems Analysis team at CATF takes an interdisciplinary approach to illustrating robust, risk-informed, feasible energy system transition pathways. The objective of the program is to systematically address the modeling limitations highlighted above, and to root CATF’s programmatic efforts within a framework of economic, socio-political, and institutional feasibility.